Automated decision support systems in healthcare are fundamentally enhancing diagnostic and treatment processes. By harnessing machine learning and advanced algorithms, these systems provide actionable insights at the point of care, supporting clinicians in making accurate, data-driven decisions. The ability to deliver precise, evidence-based guidance at each step is transforming patient outcomes and healthcare efficiency.
Understanding the Role of Clinical Decision Support Tools in Modern Healthcare
Clinical decision support (CDS) tools are an essential component of modern healthcare, enhancing the decision-making process for healthcare providers by offering valuable, timely information. Here are five reasons these tools have garnered widespread attention:
Improved Diagnostic Accuracy: CDS tools, especially when integrated with electronic health records (EHRs), provide clinicians with patient-specific data, helping to identify potential conditions early and accurately.
Reduction in Medical Errors: By alerting healthcare providers to potential drug interactions and contraindications, CDS systems reduce the risk of adverse events, thus safeguarding patient safety.
Enhanced Workflow Efficiency: With computerized decision support, clinicians can reduce time spent on data retrieval and focus on patient care, streamlining clinical workflows.
Data-Driven Personalized Care: CDS tools allow for individualized treatment plans based on clinical data, which can adjust recommendations in real-time as new information becomes available.
Evidence-Based Recommendations: These systems draw from a vast database of clinical guidelines and research findings, giving healthcare providers insights grounded in the latest medical evidence.
The Value of Decision Support Systems for Improving Patient Outcomes
Clinical decision support systems are vital in improving patient outcomes, as they bring structured, evidence-based insights directly into clinical workflows. By supporting clinicians in making informed decisions, these tools enhance diagnostic accuracy and enable timely interventions and personalized care.
Using Drug Interaction Alerts to Prevent Adverse Reactions
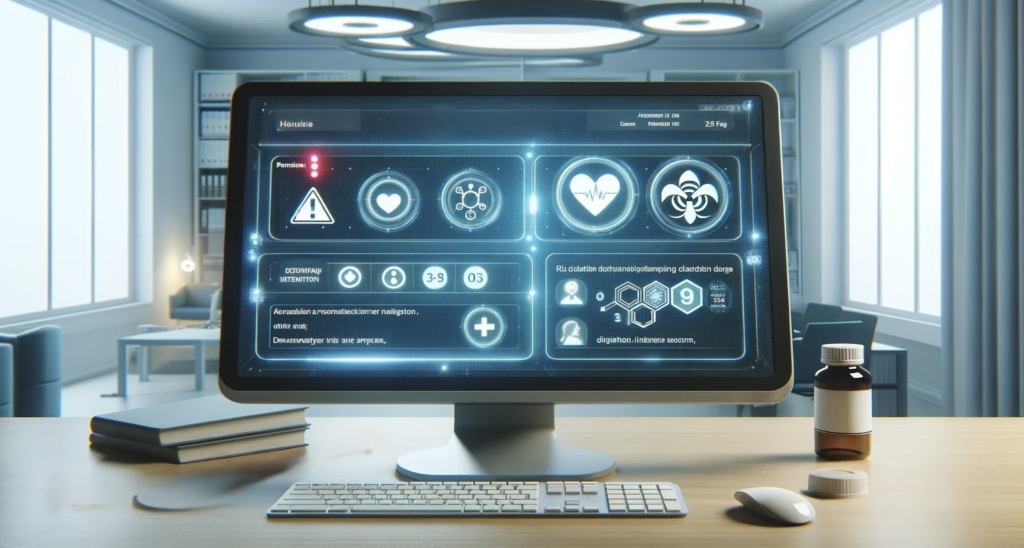
Preventing adverse drug interactions is one of the most practical applications of computerized clinical decision support systems. By integrating with electronic health record systems, these tools use clinical data to identify and alert healthcare providers about potential risks when prescribing new medications. This functionality ensures clinicians can take immediate action to prevent harmful outcomes.
A study published in International Journal of Medical Informatics highlighted how clinical decision support interventions reduce medication-related errors by flagging dangerous drug interactions in real-time [1]. These systems provide actionable insights directly within the clinical workflow, helping healthcare professionals avoid prescription errors. With the use of computerized provider order entry systems, such alerts improve both medication safety and overall patient care. By embedding these features into healthcare settings, clinicians ensure safer prescriptions and enhanced patient outcomes.
Enhancing Diagnostic Precision with AI-Driven Symptom Checkers
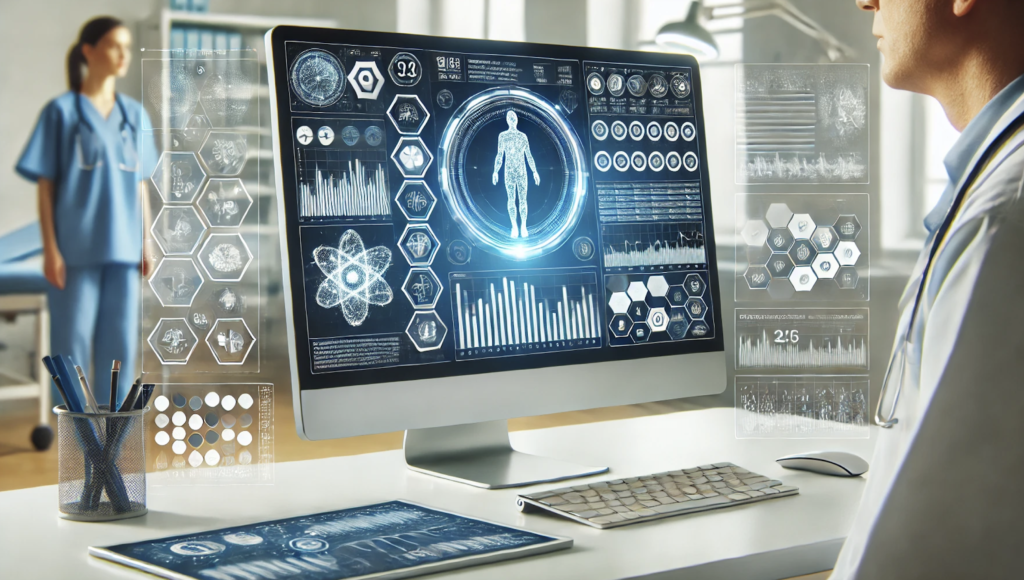
AI-powered diagnostic support tools are reshaping the way healthcare providers approach initial assessments. These computerized decision support systems analyze patient-reported symptoms alongside their medical history and match them with possible conditions using advanced algorithms. This innovation reduces diagnostic errors and ensures evidence-based recommendations during clinical decision-making.
Research from the Journal of Personalized Medicine demonstrated that computer-based clinical decision support systems equipped with AI symptom checkers improved diagnostic precision [2]. These tools integrate seamlessly into electronic health record systems, allowing clinicians to access targeted diagnostic suggestions at the point of care. By incorporating clinical practice guidelines into their analysis, these tools provide effective clinical decision support, improving both efficiency and patient outcomes in diverse healthcare environments.
Personalizing Patient Care with Risk Stratification Models
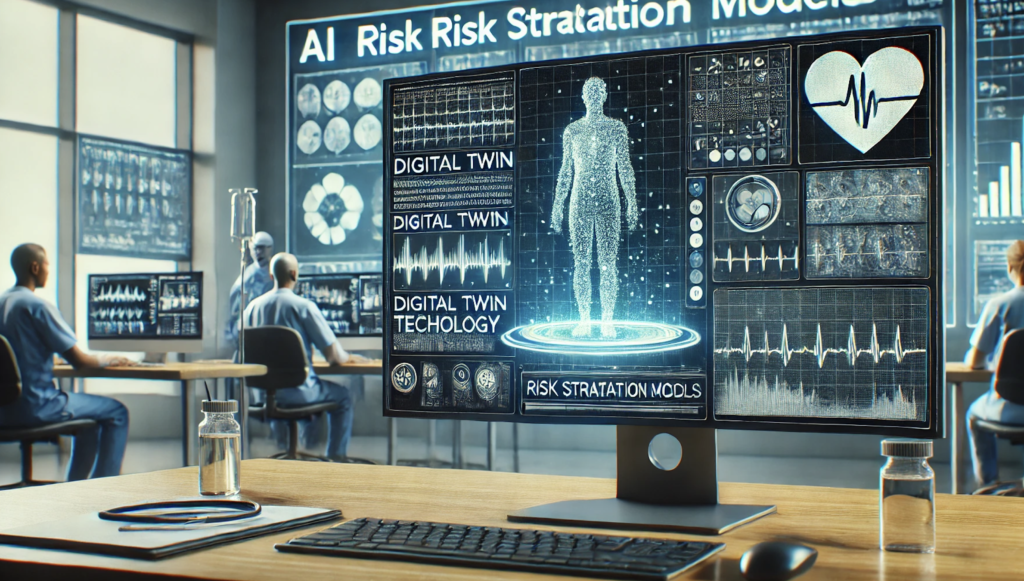
Preparing for a Future with Advanced Decision Support Technology
As healthcare continues to integrate advanced technology, the potential for clinical decision support systems to evolve is vast. Future CDS tools will likely leverage more sophisticated machine learning algorithms, enabling continuous improvement as the systems learn from accumulating patient data. Real-time data integration from multiple sources, including wearable devices and remote monitoring systems, is expected to enhance decision-making accuracy by providing clinicians with a fuller picture of the patient’s health status.
The development of interoperable health information technology systems will also enable a seamless exchange of clinical data across different healthcare settings. This interconnectivity will enhance the effectiveness of CDS tools, allowing healthcare providers to access consistent, comprehensive data regardless of the facility. As AI and machine learning models evolve, CDS systems will continue to offer increasingly nuanced insights, supporting healthcare professionals in making data-driven clinical decisions.
Get in Touch to Transform Your Health Practice with AI-Driven Clinical Support
Contact Neural Board to explore AI advisory services tailored to enhance patient care and improve decision-making in clinical settings. Our expertise in healthcare AI can support your practice in integrating advanced decision support tools effectively.
References
Pirnejad, Habibollah, et al. “Preventing potential drug-drug interactions through alerting decision support systems: a clinical context based methodology.” International journal of medical informatics 127 (2019): 18-26.
Wiedermann, Christian J., et al. “Redesigning primary care: the emergence of artificial-intelligence-driven symptom diagnostic tools.” Journal of Personalized Medicine 13.9 (2023): 1379.
Mohsin, S. N., et al. “The role of artificial intelligence in prediction, risk stratification, and personalized treatment planning for congenital heart diseases. Cureus. 15 (8), e44374 (2023).” 2023.