Artificial intelligence in radiology transforms complex data into intuitive, actionable insights. AI algorithms analyze vast amounts of imaging data, improving diagnostic accuracy and streamlining workflows. This article explores how AI systems enhance decision-making, optimize patient care, and contribute to the evolution of radiology practice.
Understanding AI’s Role in Modern Radiology
AI in the field of radiology is designed to assist clinicians by automating routine tasks, improving diagnostic accuracy, and accelerating workflow efficiency. The European Society of Radiology has recognized the impact of artificial intelligence, emphasizing its role in enhancing clinical decision-making.
Five key reasons for the growing adoption of AI:
Increased diagnostic accuracy: AI algorithms detect subtle patterns in radiological images, reducing human error and improving cancer detection rates.
Enhanced workflow efficiency: AI-powered automation helps radiologists manage high volumes of medical imaging data, ensuring faster reporting and better patient outcomes.
Integration of artificial intelligence with precision medicine: AI solutions analyze patient data from various sources, enabling more personalized treatment approaches.
Reduction of radiologist workload: AI applications in radiology handle repetitive tasks, allowing specialists to focus on complex cases.
Improved explainability of artificial intelligence: Advancements in explainable artificial intelligence (XAI) ensure that AI models provide transparent, interpretable results.
Why AI is Essential for Advancing Radiology
The increasing demand for timely and accurate diagnoses makes AI a crucial component of modern radiology. By reducing errors and accelerating medical image analysis, artificial intelligence improves patient care and ensures better treatment outcomes. AI tools not only enhance the efficiency of radiology workflows but also support radiologists in making more informed clinical decisions. The integration of AI into radiology bridges the gap between advanced technology and human expertise, leading to significant improvements in healthcare.
AI for Detecting Musculoskeletal Disorders in MRI and X-Ray Imaging
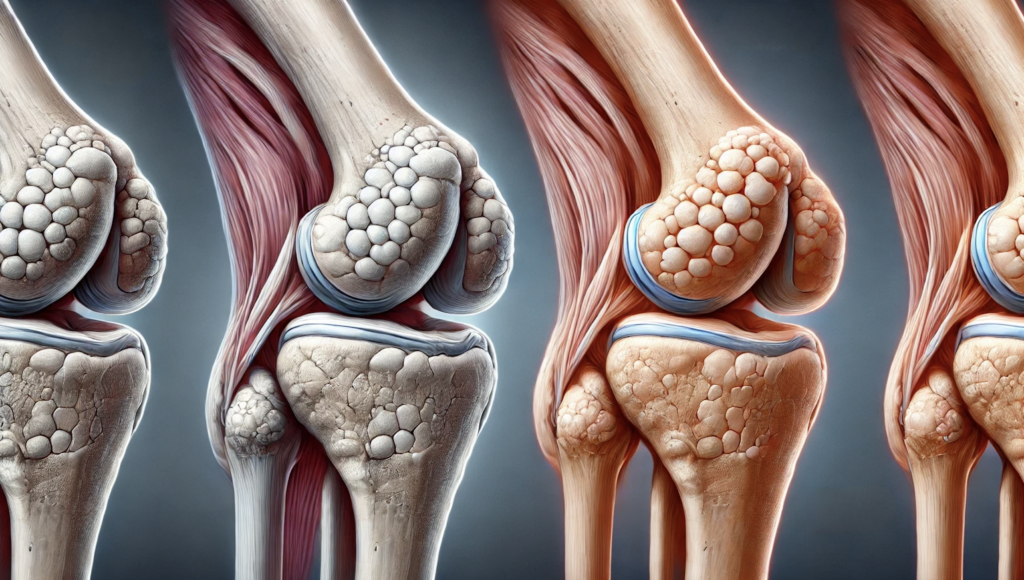
The article by Gitto et al. (2024) [1] provides a detailed review of AI applications in musculoskeletal imaging, focusing on the detection, classification, and prognosis of musculoskeletal disorders. AI systems enhance trauma imaging by accurately detecting fractures, particularly in high-pressure emergency settings. Large datasets, such as MURA, have been used to train sophisticated AI algorithms for recognizing abnormalities in CT images, X-rays, and MRI scans.
For bone age estimation, AI applications automate assessments based on traditional methods like Greulich-Pyle and Tanner-Whitehouse, improving efficiency while reducing variability. AI also supports osteoarthritis diagnosis, integrating imaging biomarkers with clinical data to predict disease progression and aid early intervention. In musculoskeletal oncology, AI-driven models assist in tumor classification and prognosis, improving radiology AI applications. Additionally, AI solutions optimize orthopedic implant evaluations, helping to detect complications and assess positioning accuracy. The study highlights that while AI can enhance radiologists’ capabilities, successful AI integration into clinical settings requires further validation and standardization.
AI-Assisted MRI Interpretation for Early Detection of Neurodegenerative Diseases
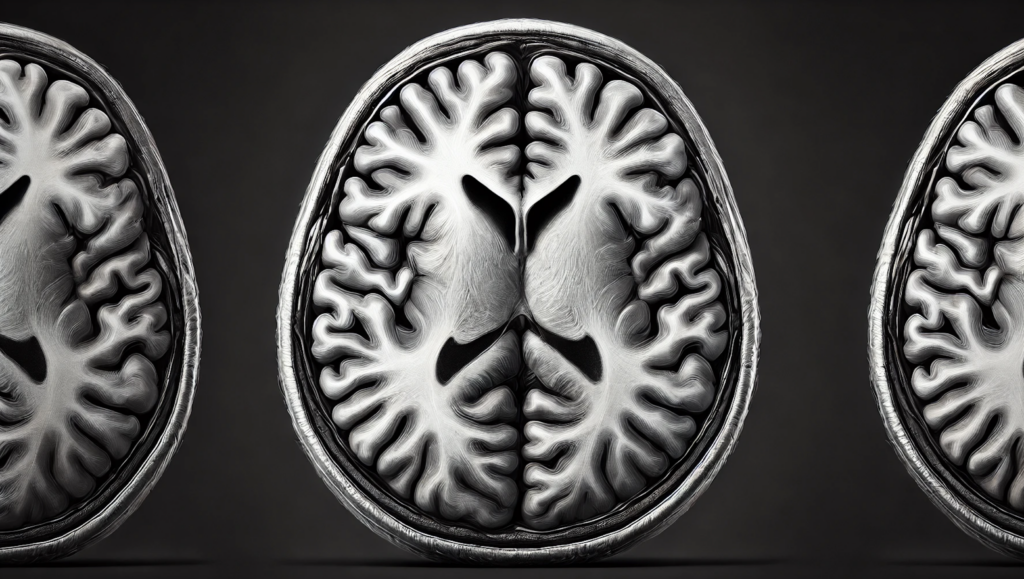
The study by Ali (2022) [2] explores AI applications in neurodegenerative disease research, focusing on early detection, cognitive decline prediction, and biomarker identification. AI use in neurology analyzes multimodal medical imaging data, including MRI, PET, and EEG, to identify subtle brain abnormalities. Artificial intelligence in medicine enhances differentiation between overlapping neurological conditions, increasing diagnostic specificity and improving collaboration between radiologists and AI developers.
Beyond imaging, AI technologies track cognitive decline using speech, gait, and behavioral pattern analysis, improving non-invasive disease monitoring. AI-powered wearable devices provide real-time assessments, supporting early intervention strategies. Explainable artificial intelligence enables transparency in AI decision-making processes, helping clinicians interpret findings. The impact of artificial intelligence extends to optimizing clinical trials by accelerating patient recruitment and predicting drug responses. However, challenges like data heterogeneity and regulatory concerns must be addressed to ensure broad AI integration in radiology.
AI-Based Automated Fracture Detection in X-Rays
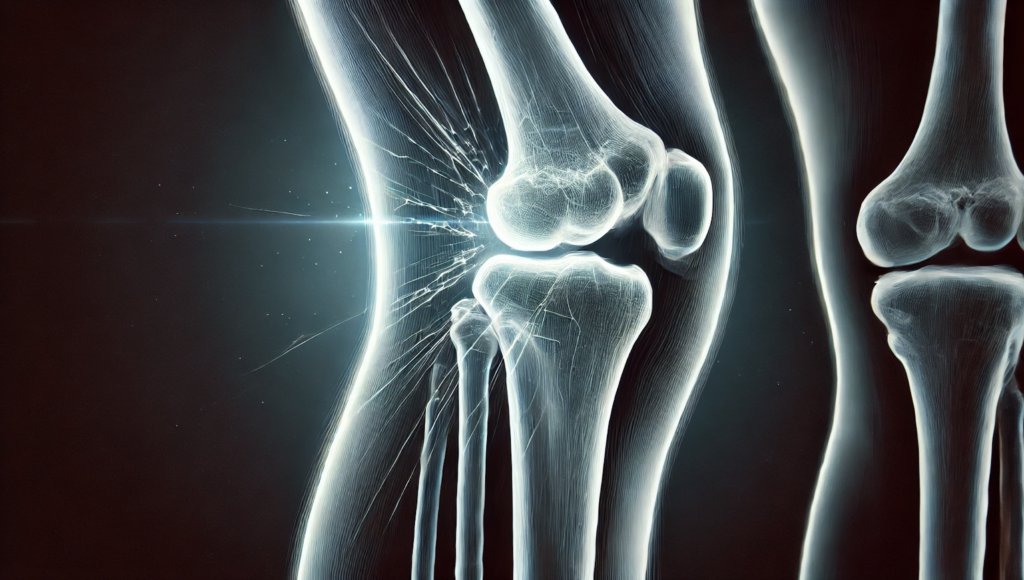
The article by Sharma (2023) [3] highlights the role of artificial intelligence for fracture diagnosis in orthopedic X-rays. Traditional fracture diagnosis relies on manual interpretation, which is time-consuming and prone to errors. AI systems based on artificial neural networks and deep learning models have been trained on vast datasets to improve fracture detection accuracy.
AI applications in radiology leverage convolutional neural networks (CNNs) to identify fractures with accuracy comparable to expert radiologists. AI can classify fracture severity, guiding treatment decisions and improving patient outcomes. Natural language processing (NLP) further enhances AI capabilities by extracting key insights from radiology reports, integrating textual and imaging data for a more comprehensive diagnosis.
Advancements in AI-based fracture detection incorporate CT images and MRI scans, refining model precision. AI integration into radiology automates routine tasks, prioritizing urgent cases and accelerating workflows. Challenges such as regulatory oversight and ethical considerations must be addressed for safe and effective implementation of AI in radiology.
The Future of AI in Radiology: What’s Next?
AI-driven advancements in radiology continue to evolve, shaping the future of medical imaging and clinical decision-making. The adoption of AI into clinical practice is accelerating, with new AI models offering greater accuracy in detecting complex conditions. However, regulatory and ethical challenges remain, requiring a structured approach to ensure responsible implementation.
One of the most promising areas is the expansion of deep learning models that improve radiology AI applications by providing faster and more accurate analyses. AI technologies are being refined to offer real-time clinical support, helping radiologists prioritize cases based on urgency and detect abnormalities with greater precision. This contributes to reducing workloads while improving overall efficiency in radiology departments.
Explainable artificial intelligence is becoming a critical component, ensuring that AI-generated results remain interpretable and reliable for clinical use. Collaboration between radiologists, AI developers, and regulatory bodies is key to integrating AI into radiology while maintaining transparency in AI decision-making processes. Future AI solutions will likely emphasize personalized medicine, integrating imaging data with genomic and clinical data to offer more tailored treatment approaches.
Despite advancements, achieving successful AI integration in radiology requires overcoming concerns about bias, validation, and physician acceptance. The field will continue to evolve as interdisciplinary efforts focus on standardizing AI systems to meet the growing demands of modern healthcare.
Contact Us for AI Integration in Healthcare
AI is transforming radiology, and implementing AI solutions can enhance diagnostic accuracy and efficiency. Our expertise in AI-driven healthcare solutions ensures seamless AI integration into radiology. Whether optimizing medical imaging workflows or developing AI applications for radiology, we provide tailored solutions that align with your healthcare needs. Reach out to explore how AI can enhance your radiology practice.
References
Gitto, Salvatore, et al. “AI applications in musculoskeletal imaging: a narrative review.” European Radiology Experimental 8.1 (2024): 22.
Ali, Hassan. “AI in neurodegenerative disease research: Early detection, cognitive decline prediction, and brain imaging biomarker identification.” Int J Eng Technol Res Manag 6.10 (2022): 71.
Sharma, Sanskrati. “Artificial intelligence for fracture diagnosis in orthopedic X-rays: current developments and future potential.” SICOT-J 9 (2023): 21.