Artificial intelligence (AI) is transforming drug discovery and development, offering new ways to optimize drug design, enhance clinical trial design, and improve drug safety. With AI-driven models, pharmaceutical companies can predict drug efficacy, accelerate research, and reduce costs. AI in the pharmaceutical industry continues to push boundaries, integrating data-driven insights with deep learning methods to advance precision medicine.
Understanding the Role of AI in Drug Discovery and Development
AI-driven approaches are reshaping the drug discovery process by leveraging deep learning for drug design and repurposing. The use of artificial intelligence enables faster and more efficient identification of potential drug candidates, cutting down the time needed for new drug approvals.
Accelerated drug target identification – AI-powered tools enhance the prediction of drug interactions, reducing reliance on traditional screening methods.
Generative AI for de novo drug design – AI creates molecular structures with desired pharmacological properties, improving computer-aided drug design.
Enhanced drug repositioning – AI applications help identify new drug candidates by analyzing existing compounds for new therapeutic uses.
Optimized clinical trial design – AI-driven models refine trial protocols and predict patient responses.
Improved drug safety assessments – Explainable artificial intelligence ensures transparency in risk evaluation.
Why AI is Essential for the Future of Drug Development
The use of AI in drug development enhances efficiency by integrating artificial intelligence technologies into every stage of the drug development process. AI reduces costs, improves drug efficacy, and enables personalized medicine.
AI for Protein Structure Prediction and Drug Target Identification
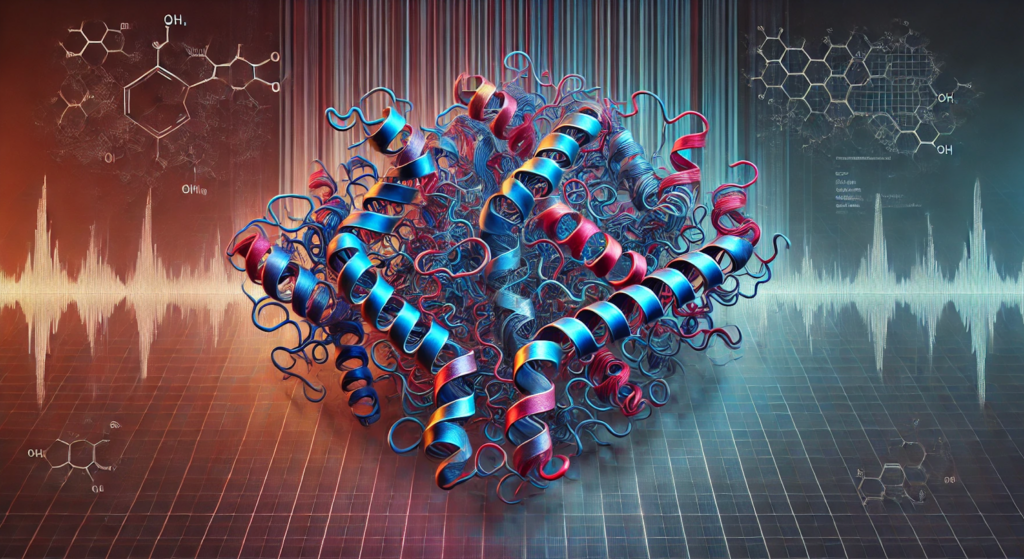
Traditional methods like X-ray crystallography and cryogenic electron microscopy have been critical for understanding protein structures but are expensive and time-consuming. The introduction of AI-driven protein structure prediction models [1], such as AlphaFold2, ESMFold, and RoseTTAFold, has transformed the drug discovery process by allowing accurate structure predictions from amino acid sequences. These models differ in methodology—AlphaFold2 relies on multiple sequence alignments, while ESMFold uses a transformer-based approach to accelerate predictions.
AI is also instrumental in predicting protein-protein interactions, which helps in identifying drug compounds targeting complex molecular mechanisms. The ability to model protein conformations enables AI to enhance ligand docking and facilitate de novo drug design. AI-driven tools such as ProteinMPNN and EvoDiff assist in designing potential drug candidates tailored for specific therapeutic needs, improving precision in molecular design.
Despite its advantages, challenges persist, particularly in predicting ligand-induced structural changes. The computational demands of AI-based structure prediction models require high-resource environments, limiting accessibility. However, ongoing advancements in deep learning algorithms and cloud-based AI platforms continue to improve the scalability of AI applications in drug discovery programs.
Machine Learning in Drug Repurposing for Rare Diseases
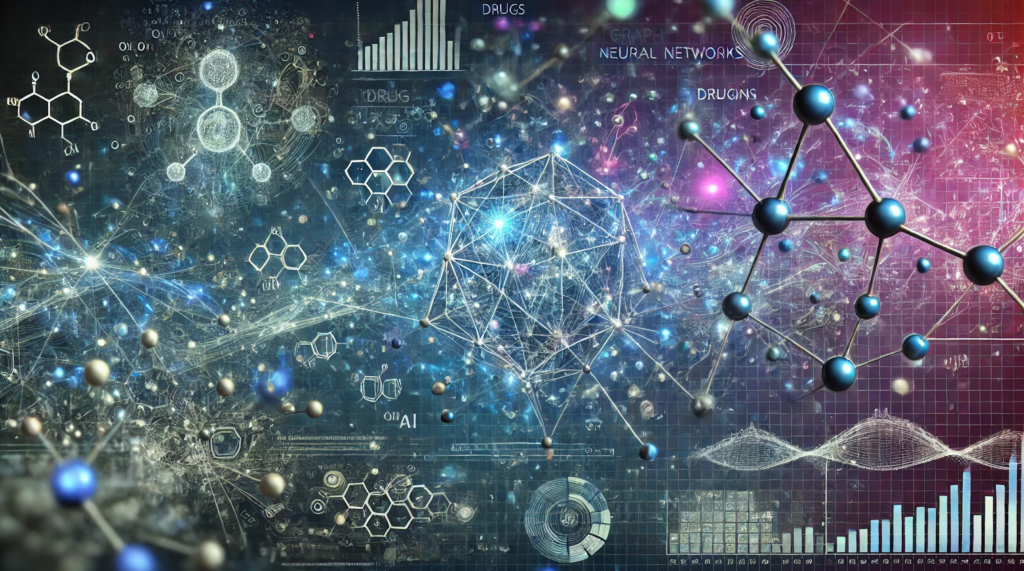
AI-driven drug repositioning [2] offers a cost-effective alternative to traditional drug discovery, particularly for rare diseases where research funding is limited. Machine learning algorithms analyze vast pharmacological datasets to identify existing drugs that may be repurposed for new therapeutic applications. These algorithms utilize supervised learning models, such as neural networks and random forests, alongside unsupervised learning techniques, including clustering and dimensionality reduction.
One key AI approach in drug discovery efforts is the use of graph neural networks (GNNs) to model drug-target interactions, helping uncover hidden therapeutic potential. Virtual screening and molecular docking simulations further enhance drug repurposing, enabling rapid evaluation of potential drug candidates.
Successful AI-driven drug repurposing cases include thalidomide, initially developed as a sedative but later repurposed for multiple myeloma and leprosy. Similarly, sildenafil, intended for hypertension, became a widely used treatment for erectile dysfunction. AI has also identified new drug candidates for infectious diseases, including baricitinib for COVID-19.
Despite promising results, AI-driven drug repurposing faces challenges, such as regulatory barriers, data quality issues, and interpretability concerns. Moving forward, improved AI transparency, interdisciplinary collaboration, and enhanced real-world data integration will be essential to advancing the field of artificial intelligence-enabled discovery.
Artificial Intelligence for Clinical Trial Optimization and Patient Recruitment
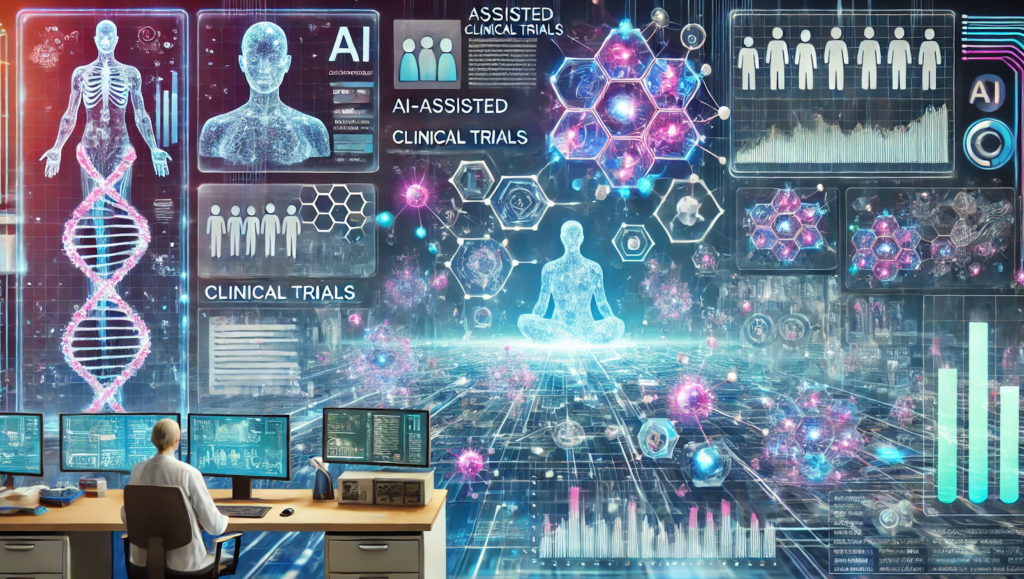
Patient recruitment inefficiencies contribute to costly delays in clinical trial design [3]. AI-based recruitment models improve patient matching by analyzing medical records, trial criteria, and historical data. Machine intelligence optimizes recruitment through hybrid classification models, integrating autoencoders for feature extraction and ensemble learning techniques, including logistic regression, decision trees, and support vector machines.
Beyond ML, game-theoretic approaches help balance the interests of stakeholders—patients, doctors, and research firms—ensuring strategic recruitment. AI models predict patient eligibility with high accuracy, streamlining trial design and improving enrollment efficiency.
Simulation studies validate AI-driven strategies, demonstrating enhanced recruitment success rates. Future AI-driven trial design strategies will include real-time patient engagement platforms and adaptive recruitment protocols tailored to dynamic trial needs. The integration of deep learning methods with real-world clinical data will further optimize participant selection, paving the way for deep learning-guided discovery in drug development efforts.
The Future Impact of AI in Drug Discovery and Development
The application of artificial intelligence in pharma will continue to expand. Advancements in deep learning algorithms and multiproperty-driven molecular discovery are expected to reshape the field of drug development.
AI-driven techniques will enhance sequence-based drug design, utilizing using deep neural networks for rethinking drug design and optimizing identification of potential drug candidates. Multimodal biomedical AI will integrate genomic, proteomic, and clinical data to refine predictions for prediction of drug efficacy and safety.
AI-driven innovations are expected to impact several areas:
Key Area | Expected Impact |
---|---|
AI-powered trial simulations | Virtual patient modeling for enhanced clinical trial design. |
Explainable AI models | Improved transparency and validation in AI-driven research. |
Deep learning-guided discovery | AI-driven de novo drug design for tailored therapeutics. |
Integration of AI tools | Real-time AI analytics in drug screening and validation. |
AI will continue to transform the drug discovery process, but challenges such as regulatory acceptance, data standardization, and ethical considerations remain. Ensuring the responsible use of AI will be crucial to its success in the drug development process.
Contact Us for AI Integration in Healthcare and Drug Development
Pharmaceutical companies can benefit from incorporating AI into drug discovery programs. AI tools enhance the entire drug development pipeline, improving precision and efficiency. Contact us to explore opportunities and challenges in AI-driven drug discovery.
References
Qiu, Xinru, et al. “Advances in AI for protein structure prediction: implications for cancer drug discovery and development.” Biomolecules 14.3 (2024): 339.
Islam, Md Tajul, et al. “Ai-Driven Drug Repurposing: Uncovering Hidden Potentials Of Established Medications For Rare Disease Treatment.” Library of Progress-Library Science, Information Technology & Computer 44.3 (2024).
Kumar, CU Om, Isshaan Singh, and M. Suguna. “Optimizing Patient Recruitment for Clinical Trials: A Hybrid Classification Model and Game-Theoretic Approach for Strategic Interaction.” IEEE Access 12 (2024): 10254-10280.