Deep learning is reshaping the future of healthcare by enabling precise diagnosis, personalized treatment, and improved patient outcomes. Through deep neural networks and advanced learning algorithms, artificial intelligence is driving innovations in medical image analysis, electronic health records (EHR), and predictive analytics, creating a more efficient healthcare system.
Overview: How Deep Learning is Revolutionizing Medicine and Healthcare
Deep learning in healthcare is gaining traction for several compelling reasons, bringing advanced technologies like artificial neural networks and deep models into everyday clinical applications. Here are five key reasons for its growing importance:
Improved Accuracy in Diagnosis: Deep learning algorithms, particularly convolutional neural networks (CNNs), are outperforming traditional machine learning methods in tasks like medical image analysis. Studies have shown deep learning models can achieve human-level accuracy in diagnosing conditions from X-rays and MRIs.
Personalized Treatment Plans: AI-powered systems analyze vast datasets, including patient history, genetics, and medical images, to create customized treatment recommendations. This allows healthcare providers to offer more targeted therapies.
Enhanced Predictive Analytics: By using deep learning models, healthcare professionals can predict patient outcomes and potential complications with higher accuracy. Predictive models based on deep learning are being used for early detection of diseases like cancer and heart disease.
Efficient Data Analysis: Deep learning approaches, such as representation learning and unsupervised learning, enable the efficient handling of large amounts of healthcare data, reducing the workload for clinicians while improving decision-making accuracy.
Integration with Electronic Health Records: Deep learning-based systems are being used to analyze and optimize EHR data, making it easier for healthcare providers to manage patient information and deliver better care.
The Importance of AI and Deep Learning in Modern Healthcare
Artificial intelligence, especially through deep learning methods, is revolutionizing healthcare. Below are just a few examples of how deep learning is being applied in real-world healthcare systems, from EHRs to predictive analytics, showing its transformative power.
Machine Learning and Deep Learning in Electronic Health Records (EHR)
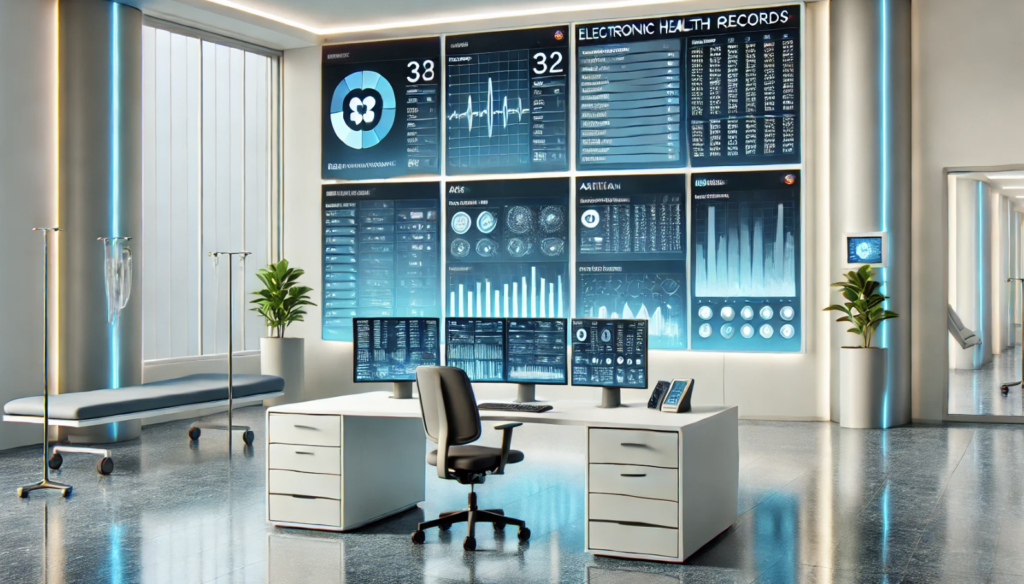
The application of deep learning in healthcare has found significant success in enhancing the use of electronic health records (EHRs). Traditional EHR systems store vast amounts of healthcare data but often struggle with data accessibility and integration. Deep learning methods help solve these issues by efficiently handling unstructured data and identifying meaningful patterns that are not easily visible through traditional methods.
According to a study published in npj Digital Medicine [1], deep learning models have proven effective in identifying personal and family histories of suicidal thoughts and behaviors (PSH and FSH) from electronic health records (EHRs). The research employed advanced transformer-based models like Bio_ClinicalBERT and GatorTron, which significantly outperformed traditional rule-based approaches in detecting these critical risk factors. The deep learning models processed complex clinical notes, which often contained unstructured data, leading to more accurate identification of patients at high risk for suicide. By utilizing deep learning, healthcare providers can uncover vital mental health information that is frequently missed in structured EHR data. This enables improved stratification and more informed decision-making, ultimately helping to identify and intervene in cases that might otherwise go unnoticed
The Role of Deep Learning in Medical Image Analysis
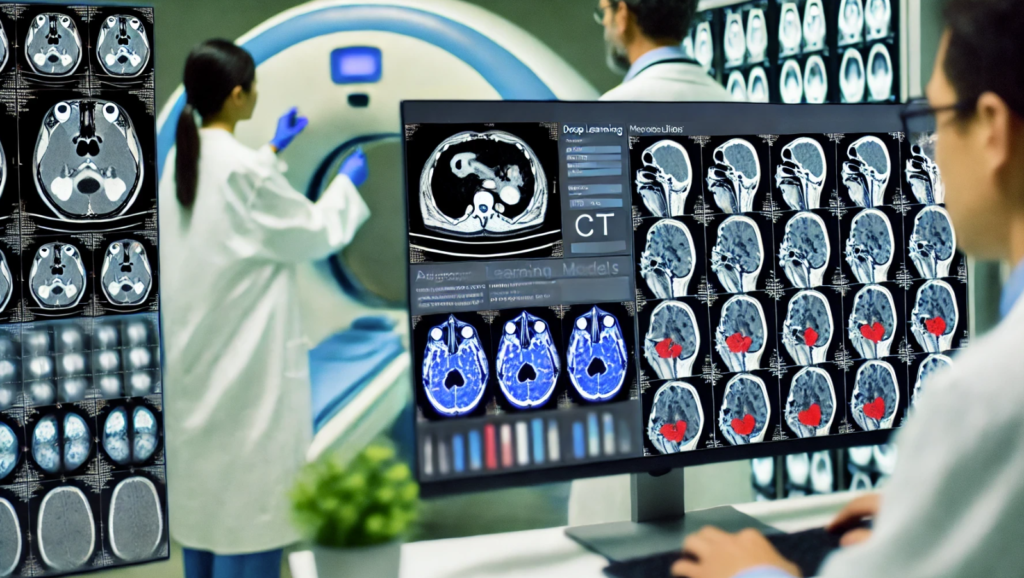
Medical image analysis is one of the most successful applications of deep learning in healthcare. Deep convolutional neural networks (CNNs) have proven to be highly effective in classifying, segmenting, and detecting anomalies in medical images such as MRI, CT scans, and X-rays. One remarkable example comes from a study on brain tumor classification using a transfer learning, which demonstrated that deep learning models can significantly outperform traditional machine learning techniques.
According to the comparative analysis [2] conducted in the study on MRI-based brain tumor classification using transfer learning, deep learning models like DenseNet121 outperformed other pre-trained models such as ResNet50 and InceptionV3 in terms of accuracy and robustness. These findings are crucial for healthcare professionals, as faster and more accurate diagnoses significantly improve patient outcomes, particularly for time-sensitive conditions like brain tumors. Transfer learning’s ability to fine-tune pre-trained models on specific healthcare tasks makes it particularly promising, especially in scenarios where large, labeled medical datasets are scarce.
Application of Deep Learning for Predictive Analytics in Healthcare
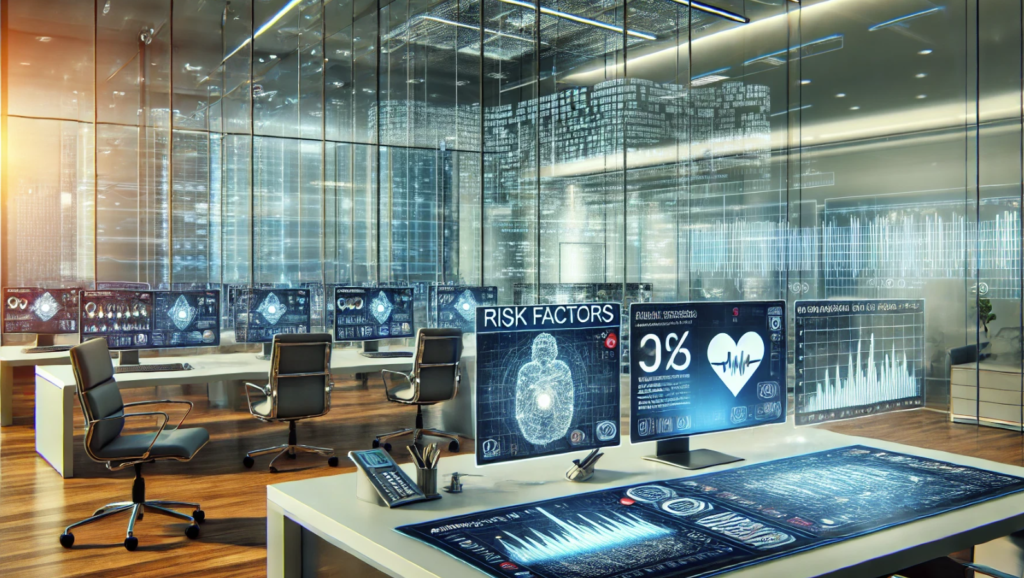
Predictive analytics is a rapidly growing field in healthcare and deep learning models play a pivotal role in its advancement. With predictive analytics, AI models analyze past data to predict future events, such as disease outbreaks, patient deterioration, or the success of certain treatments.
According to a study published in the Journal of Electrical Systems and Information Technology [3], deep learning models have shown great potential in predicting healthcare outcomes by analyzing vast amounts of healthcare data. These models are capable of forecasting events such as disease progression, hospital readmissions, and mortality rates by processing diverse data sources, including clinical notes, lab results, and imaging data. The study highlights that deep learning algorithms can efficiently manage and interpret this complex data, providing more accurate predictions than traditional methods. As these models continue to evolve, they offer a transformative solution for predictive analytics in healthcare, improving clinical decision support and helping healthcare professionals make informed decisions that enhance patient outcomes
Deep learning in predictive analytics allows healthcare systems to be more proactive, identifying high-risk patients early and allocating resources more efficiently. As the application of deep learning continues to evolve, predictive analytics will become a core part of patient care and hospital management.
The Future of Healthcare: Why AI and Deep Learning Will Drive Innovation
The future of healthcare will be shaped by the continued integration of machine learning models and deep learning systems. However, there are challenges that need to be addressed to fully unlock the potential of deep learning in healthcare. One significant challenge is the need for more diverse and high-quality datasets to train deep learning algorithms. Without enough representative data, AI models risk producing biased or inaccurate results. Privacy concerns also play a critical role, as patient data must be protected while ensuring that AI systems remain effective.
On the other hand, the opportunities for deep learning-based systems in healthcare are vast. AI-driven innovations will not only enhance the precision of diagnostics and treatment plans but also reduce the burden on healthcare professionals by automating routine tasks. As advances in deep learning architecture and unsupervised learning continue, the ability to analyze complex medical data in real time will redefine the healthcare landscape.
In the coming years, we can expect more clinically applicable deep learning models to be adopted, improving patient outcomes and operational efficiencies across healthcare systems globally.
Leverage AI and Deep Learning to Enhance Your Healthcare Solutions
If you’re looking to implement AI and deep learning solutions in your healthcare system, contact us at Neural Board. We provide expert advisory services to help you integrate cutting-edge AI technologies into your practice.
References:
- Adekkanattu, Prakash, et al. “Deep learning for identifying personal and family history of suicidal thoughts and behaviors from EHRs.” npj Digital Medicine 7.1 (2024): 260.
- Poudyal, Anil, Niruta Devkota, and Gayatri Budha. “MRI-based Brain Tumor Classification using Transfer Learning: A Comparative Analysis.” International Journal of Computer Applications 975: 8887.
- Badawy, Mohammed, Nagy Ramadan, and Hesham Ahmed Hefny. “Healthcare predictive analytics using machine learning and deep learning techniques: a survey.” Journal of Electrical Systems and Information Technology 10.1 (2023): 40.
Q: What is a deep belief network, and how is it used in healthcare?
A: A deep belief network is a type of deep learning model used for unsupervised learning. In healthcare, it can be applied to analyze complex datasets, uncover hidden patterns in patient data, and improve predictive modeling in various medical applications.
Q: How does supervised learning differ from unsupervised learning in the context of healthcare?
A: Supervised learning in healthcare uses labeled data to train models that can predict outcomes, while unsupervised learning identifies patterns in unlabeled data. Both approaches can be applied in different scenarios, such as patient outcome predictions or clustering patient populations.
Q: Can you explain the significance of validation of a deep learning model in healthcare?
A: Validation of a deep learning model is crucial in healthcare to ensure its accuracy, reliability, and applicability in real-world settings. It helps to build trust among healthcare professionals and patients, ensuring that the model’s predictions are clinically relevant.
Q: How does semi-supervised learning contribute to advancements in learning for healthcare?
A: Semi-supervised learning combines labeled and unlabeled data, making it especially beneficial in healthcare where labeled data may be scarce. This approach allows for improved model training and better performance in various healthcare applications.